The thesis defense of Mrs. Maria Gracia Collantes-Padrique was held in-person on July 3, 2024, 2:00PM at the NIGS Seminar Room. Her research is entitled “Constraining the Porosity of Geothermal Reservoir Rocks Using Petrography, Image Segmentation, and Machine Learning Classifiers” with thesis committee composing of Dr. Allan Gil S. Fernando (adviser), Dr. Maricor N. Soriano (UP-NIP, co-adviser), Mrs. Sylvia G. Ramos, M.Sc. (Premier Geo-Excel, Inc., reader), Dr. Noelynna T. Ramos (examiner) and Dr. John Dale B. Dianala (examiner).
ABSTRACT
A qualitative assessment of geothermal reservoir rock samples involves a detailed petrographic analysis. This includes visually estimating pore and vein fractions, identifying rock textures, describing the degree of alteration, identifying the hydrothermal alteration and indicated temperature, and noting the presence of shearing and various porosity types. This traditional method of visual estimation and assessment are prone to errors when averaging fields-of-view and can be labor-intensive, especially during time-sensitive drilling operations when a geologist must analyze hundreds of thin sections per well under a polarizing petrographic microscope. Porosity is a crucial parameter for calculating the recoverable steam fraction from a geothermal system. To enhance the traditional visual assessment method, this thesis explores the use of machine learning classifiers combined with detailed petrography to constrain the porosity of reservoir rocks from an active geothermal field in the Philippines. To constrain the porosity means to evaluate how certain rock features correlate to the different levels of porosity of the six lithologies obtained from the study area. The study employed machine learning for: (1) segmentation of 104 high-resolution scanned thin section images; (2) porosity classification of the scanned thin section images using input parameters obtained from segmentation and petrography; (3) application of convolutional neural networks (CNN) for porosity classification of over 4,000 photomicrographs and (4) development of CNN models for detecting epidote, a key mineral marker for production in Philippine geothermal fields. The models’ accuracies were evaluated using various metrics, including ANOVA Feature Importance Analysis, ROC curve, confusion matrix, F-score, precision, and recall. This study identified key features contributing to the porosity classification which are alteration intensity and vein formation; developed a high-precision model for detecting epidote in photomicrographs with near perfect to perfect true positive rate scores; and proposed a recommended workflow for geothermal petrology.
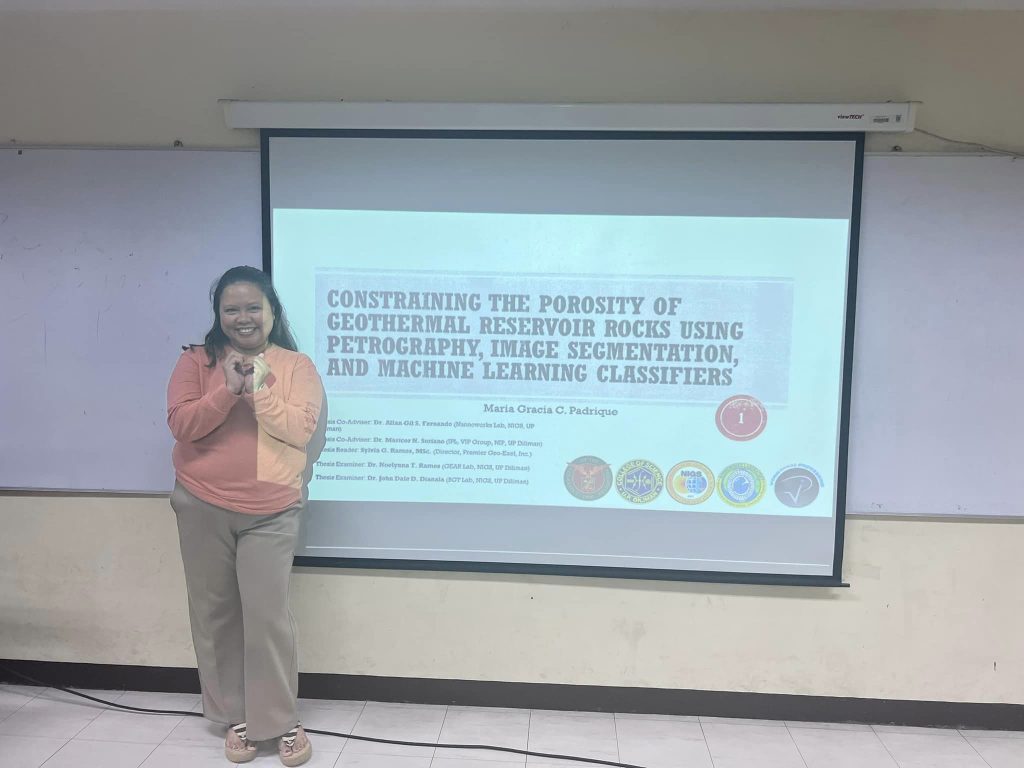
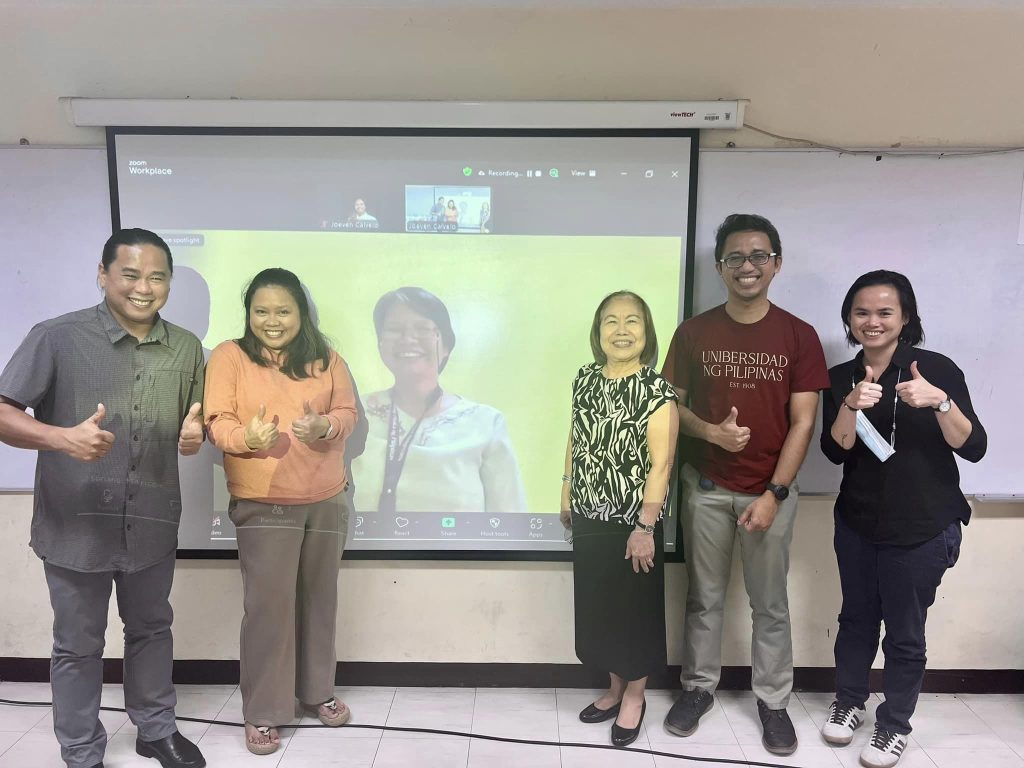
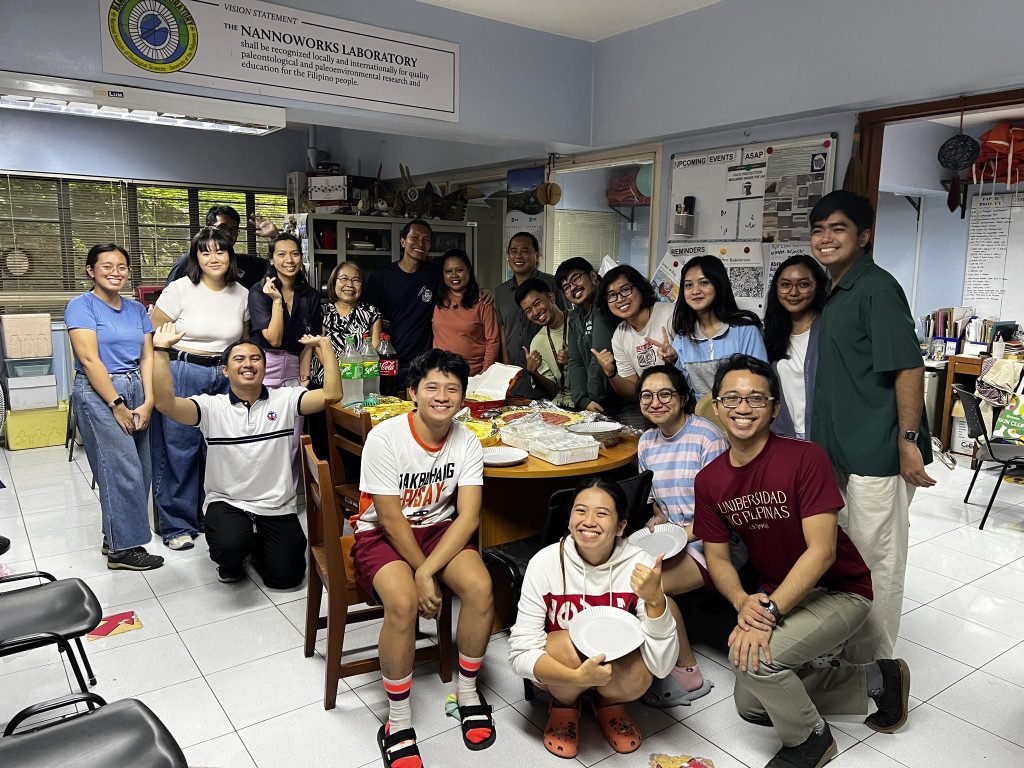